Selected Papers by Topic
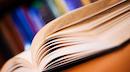
A more complete and searchable list of papers is also available.
Graph Queries
Schema-Based Query Optimisation for Graph Databases. Proc. ACM on Management of Data., 2025 (SIGMOD'25). ![]() |
Abstract: Recursive graph queries are increasingly popular for extracting information from interconnected data found in various domains such as social networks, life sciences, and business analytics. Graph data often come with schema information that describe how nodes and edges are organized. We propose a type inference mechanism that enriches recursive graph queries with relevant structural information contained in a graph schema. We show that this schema information can be useful in order to improve the performance when evaluating recursive graph queries. Furthermore, we prove that the proposed method is sound and complete, ensuring that the semantics of the query is preserved during the schema-enrichment process. |
BibTeX:
@proceedings{geneves-sigmod2025, author = {Chandan Sharma and Pierre Genevès and Nils Gesbert and Nabil Layaïda}, title = {Schema-Based Query Optimisation for Graph Databases}, journal = {Proc. ACM on Management of Data.}, year = {2025}, pages = {(to appear)}, url = {https://inria.hal.science/hal-04485125} } |
Efficient Enumeration of Recursive Plans in Transformation-based Query Optimizers. Proc. VLDB Endow. Vol. 17(11), 2024 (VLDB'24). |
Abstract: Query optimizers built on the transformation-based Volcano/Cascades framework are used in many database systems. Transformations proposed earlier on the logical query dag (LQDAG) data structure, which is key in such a framework, focus only on recursion-free queries. In this paper, we propose the recursive logical query dag (RLQDAG) which extends the LQDAG with the ability to capture and transform recursive queries, leveraging recent developments in recursive relational algebra. Specifically, this extension includes: (i) the ability of capturing and transforming sets of recursive relational terms thanks to (ii) annotated equivalence nodes used for guiding transformations that are more complex in the presence of recursion; and (iii) RLQDAG rewrite rules that transform sets of subterms in a grouped manner, instead of transforming individual terms in a sequential manner; and that (iv) incrementally update the necessary annotations. Core concepts of the RLQDAG are formalized using a syntax and formal semantics with a particular focus on subterm sharing and recursion. The result is a clean generalization of the LQDAG transformation-based approach, enabling more efficient explorations of plan spaces for recursive queries. An implementation of the proposed approach shows significant performance gains compared to the state-of-the-art. |
BibTeX:
@proceedings{geneves-vldb2024, author = {Amela Fejza and Pierre Genevès and Nabil Layaïda}, title = {Efficient Enumeration of Recursive Plans in Transformation-based Query Optimizers}, journal = {Proc. VLDB Endow.}, year = {2024}, volume = {17}, number = {11}, pages = {3095--3108}, url = {https://www.vldb.org/pvldb/vol17/p3095-geneves.pdf} } |
On the Optimization of Recursive Relational Queries: Application to Graph Queries. In Proceedings of the ACM SIGMOD International Conference on Management of Data, June ACM, 2020 (SIGMOD'20). |
Abstract: Graph databases have received a lot of attention as they are particularly useful in many applications such as social networks, life sciences and the semantic web. Various languages have emerged to query graph databases, many of which embed forms of recursion which reveal essential for navigating in graphs. The relational model has benefited from a huge body of research in the last half century and that is why many graph databases rely on techniques of relational query engines. Since its introduction, the relational model has seen various attempts to extend it with recursion and it is now possible to use recursion in several SQL or Datalog based database systems. The optimization of recursive queries remains, however, a challenge. We propose mu-RA, a variation of the Relational Algebra equipped with a fixpoint operator for expressing recursive relational queries. mu-RA can notably express unions of conjunctive regular path queries. Leveraging the fact that this fixpoint operator makes recursive terms more amenable to algebraic transformations, we propose new rewrite rules. These rules makes it possible to generate new query execution plans, that cannot be obtained with previous approaches. We present the syntax and semantics of mu-RA, and the rewriting rules that we specifically devised to tackle the optimization of recursive queries. We report on practical experiments that show that the newly generated plans can provide significant performance improvements for evaluating recursive queries over graphs. |
BibTeX:
@inproceedings{geneves-sigmod2020, author = {Louis Jachiet and Pierre Genevès and Nils Gesbert and Nabil Layaïda}, title = {On the Optimization of Recursive Relational Queries: Application to Graph Queries}, booktitle = {Proceedings of the ACM SIGMOD International Conference on Management of Data}, publisher = {ACM}, year = {2020}, pages = {681--697}, doi = {10.1145/3318464.3380567} } |
Tree Logic
Efficiently Deciding Mu-Calculus with Converse over Finite Trees. ACM Transactions on Computational Logic Vol. 16(2), 2015 (TOCL'15).
[Abstract] [PDF] [doi] [ACM DL] [Website] [Implementation] [BibTeX] |
Abstract: A tree logic and an efficient solver for checking satisfiability of a formula of the logic... |
BibTeX:
@article{geneves-tocl2015, author = {Pierre Genevès and Nabil Layaïda and Alan Schmitt and Nils Gesbert}, title = {Efficiently Deciding Mu-Calculus with Converse over Finite Trees}, journal = {ACM Trans. Comput. Log.}, year = {2015}, volume = {16}, number = {2}, pages = {16}, note = {\href{https://hal.inria.fr/hal-00868722v5/document}{https://hal.inria.fr/hal-00868722v5}}, doi = {10.1145/2724712} } |
Type Systems and Static Analysis
A Logical Approach to Deciding Semantic Subtyping. ACM Transactions on Programming Language and Systems Vol. 38(1), 2015 (TOPLAS'15). (Preliminary results were presented at the 16th International Conference on Functional Programming, 2011 (ICFP'11) |
BibTeX:
@article{geneves-toplas2015, author = {Nils Gesbert and Pierre Genevès and Nabil Layaïda}, title = {A Logical Approach to Deciding Semantic Subtyping}, journal = {ACM Trans. Program. Lang. Syst.}, year = {2015}, volume = {38}, number = {1}, pages = {3}, note = {\href{https://hal.inria.fr/hal-00848023v2/document}{https://hal.inria.fr/hal-00848023v2}}, url = {http://doi.acm.org/10.1145/2812805}, doi = {10.1145/2812805} } |
On the Analysis of Cascading Style Sheets. In Proceedings of the 21st International Conference on World Wide Web, April 2012 (WWW'12). |
Abstract: We present an original method for proving the absence of errors in CSS style sheets. |
BibTeX:
@inproceedings{geneves-www2012, author = {Pierre Genevès and Nabil Layaïda and Vincent Quint}, title = {On the Analysis of Cascading Style Sheets}, booktitle = {Proceedings of the 21st World Wide Web Conference}, year = {2012}, pages = {809-818}, note = {\href{https://hal.inria.fr/hal-00643075/document}{https://hal.inria.fr/hal-00643075}}, doi = {10.1145/2187836.2187946} } |
Efficient Static Analysis of XML Paths and Types. In Proceedings of the 2007 ACM SIGPLAN Conference on Programming Language Design and Implementation, San Diego, California, USA, 2007 (PLDI'07). |
Abstract: A new logic for reasoning over finite trees is proposed. This logic currently offers the best balance known between expressivity and complexity for decidability. It is as expressive as monadic second-order logic whereas its satisfiability is shown decidable in time complexity 2^O(n) w.r.t. size n of the formula. We present an effective algorithm in practice using symbolic techniques (BDDs), and use it for the static analysis of XPath queries in the presence of regular tree types, including XPath typing. |
BibTeX:
@inproceedings{geneves-pldi2007, author = {Pierre Genevès and Nabil Layaïda and Alan Schmitt}, title = {Efficient Static Analysis of XML Paths and Types}, booktitle = {Proceedings of the 2007 ACM SIGPLAN Conference on Programming Language Design and Implementation (PLDI)}, year = {2007}, pages = {342--351}, doi = {10.1145/1250734.1250773} } |
Data Science and Artificial Intelligence
Reproduce, Replicate, Reevaluate. The Long but Safe Way to Extend Machine Learning Methods. In Thirty-Eighth AAAI Conference on Artificial Intelligence, Vancouver, Canada, 2024 (AAAI'24). |
Abstract: Reproducibility is a desirable property of scientific research. On the one hand, it increases confidence in results. On the other hand, reproducible results can be extended on a solid basis. In rapidly developing fields such as machine learning, the latter is particularly important to ensure the reliability of research. In this paper, we present a systematic approach to reproducing (using the available implementation), replicating (using an alternative implementation) and reevaluating (using different datasets) state-of-the-art experiments. This approach enables the early detection and correction of deficiencies and thus the development of more robust and transparent machine learning methods. We detail the independent reproduction, replication, and reevaluation of the initially published experiments with a method that we want to extend. For each step, we identify issues and draw lessons learned. We further discuss solutions that have proven effective in overcoming the encountered problems. This work can serve as a guide for further reproducibility studies and generally improve reproducibility in machine learning. |
BibTeX:
@inproceedings{geneves-aaai2024, author = {Luisa Werner and Nabil Layaïda and Pierre Genevès and Jérôme Euzenat and Damien Graux}, editor = {Michael J. Wooldridge and Jennifer G. Dy and Sriraam Natarajan}, title = {Reproduce, Replicate, Reevaluate. The Long but Safe Way to Extend Machine Learning Methods}, booktitle = {Thirty-Eighth AAAI Conference on Artificial Intelligence, AAAI 2024, Thirty-Sixth Conference on Innovative Applications of Artificial Intelligence, IAAI 2024, Fourteenth Symposium on Educational Advances in Artificial Intelligence, EAAI 2014, February 20-27, 2024, Vancouver, Canada}, publisher = {AAAI Press}, year = {2024}, pages = {15850--15858}, url = {https://doi.org/10.1609/aaai.v38i14.29515}, doi = {10.1609/AAAI.V38I14.29515} } |
Knowledge Enhanced Graph Neural Networks. In 10th IEEE International Conference on Data Science and Advanced Analytics, DSAA 2023, Thessaloniki, Greece, October 9-13, 2023 IEEE, 2023 (DSAA'23). |
Abstract: Graph data is omnipresent and has a wide variety of applications, such as in natural science, social networks, or the semantic web. However, while being rich in information, graphs are often noisy and incomplete. As a result, graph completion tasks, such as node classification or link prediction, have gained attention. On one hand, neural methods, such as graph neural networks, have proven to be robust tools for learning rich representations of noisy graphs. On the other hand, symbolic methods enable exact reasoning on graphs.We propose Knowledge Enhanced Graph Neural Networks (KeGNN), a neuro-symbolic framework for graph completion that combines both paradigms as it allows for the integration of prior knowledge into a graph neural network model.Essentially, KeGNN consists of a graph neural network as a base upon which knowledge enhancement layers are stacked with the goal of refining predictions with respect to prior knowledge. We instantiate KeGNN in conjunction with two state-of-the-art graph neural networks, Graph Convolutional Networks and Graph Attention Networks, and evaluate KeGNN on multiple benchmark datasets for node classification. |
BibTeX:
@inproceedings{geneves-dsaa2023, author = {Luisa Werner and Nabil Layaïda and Pierre Genevès and Sarah Chlyah}, title = {Knowledge Enhanced Graph Neural Networks}, booktitle = {10th IEEE International Conference on Data Science and Advanced Analytics, DSAA 2023, Thessaloniki, Greece, October 9-13, 2023}, publisher = {IEEE}, year = {2023}, pages = {1--10}, url = {https://doi.org/10.1109/DSAA60987.2023.10302495}, doi = {10.1109/DSAA60987.2023.10302495} } |
Scalable and Interpretable Predictive Models for Electronic Health Records. In Proceedings of the 5th IEEE International Conference on Data Science and Advanced Analytics, October IEEE, 2018 (DSAA'18). |
Abstract: We consider the problem of predicting important clinical outcomes such as inpatient mortality, based on EHR data. We develop risk prediction models that leverage the evolution of drugs served during hospital stays. We report on lessons learned through practical experiments with real EHR data from more than one million of patients admitted to US hospitals, which is, to the best of our knowledge, one of the largest such experimental study conducted so far. |
BibTeX:
@inproceedings{geneves-dsaa2018, author = {Amela Fejza and Pierre Genevès and Nabil Layaïda and Jean-Luc Bosson}, title = {Scalable and Interpretable Predictive Models for Electronic Health Records}, booktitle = {Proceedings of the 5th IEEE International Conference on Data Science and Advanced Analytics}, publisher = {IEEE}, year = {2018}, pages = {341--350}, doi = {10.1109/DSAA.2018.00045} } |